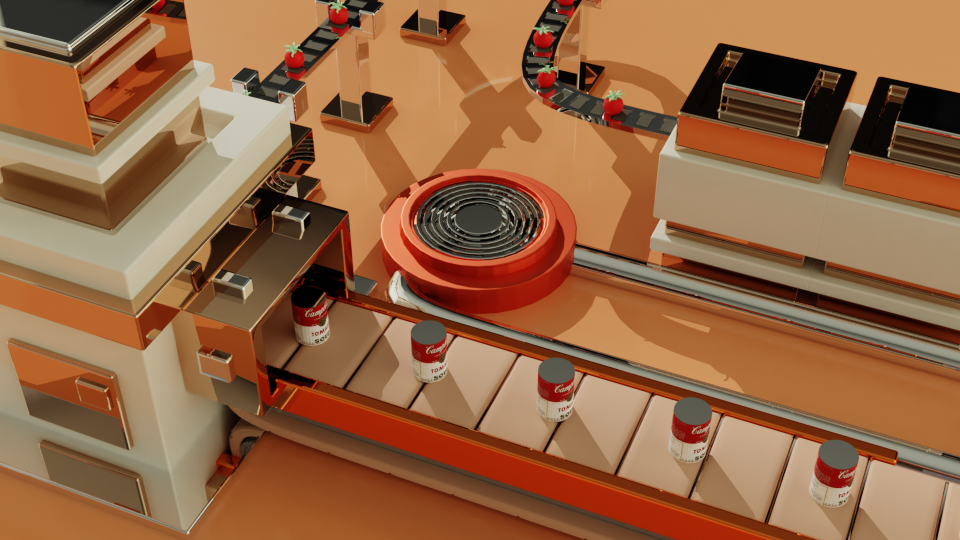
Nowadays, companies have no choice but to implement Machine Learning solutions in almost every part of their processes. This fact seems even clearer on markets where fierce competition reigns. Although Machine Learning does not redefine the company, it is certainly a powerful asset both for marketing purposes and optimization processes. It is so rooted in the companies roadmaps that now most of them rely heavily on it for the whole creation processes, from Quality Inspection of products to Public Relations.
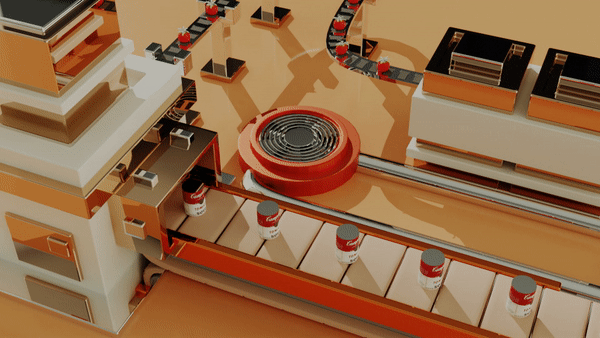
A great entity to apply Machine Learning solutions on is the Supply Chain Management (SCM). This has many reasons but the biggest is by far that SCM is in itself a complex system in which a company has a lot to lose if not handled the right way. The complexity of it is due to the diversity of actors and actions included. From suppliers to clients, everything can go wrong and destabilize the whole revenue sources of the company, allowing the competitors to benefit from these heavy mistakes.
Here are some of key aspects in which ML could improve the handling of the SCM:
QUALITY INSPECTION
Knowing that your products are good to leave the factory is always a must to prevent further losses in shipments or returned products. Although some practical tests are necessary, ML can perform evaluations of the quality of the product based on the fabrication facts. Thanks to this, the company can run tests on products susceptible of failure with knowledge of past difficulties, allowing a lighter quality inspection. It also allows the setup of a reputation table of suppliers and determine which ones are risky to work with.
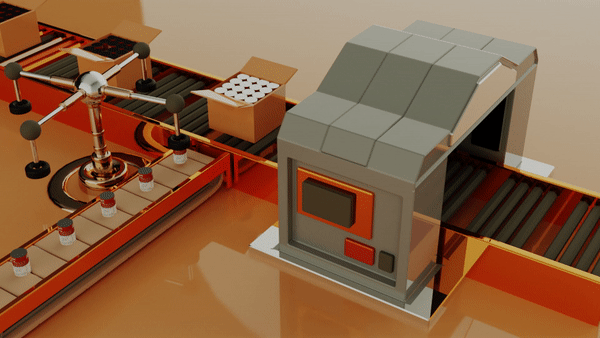
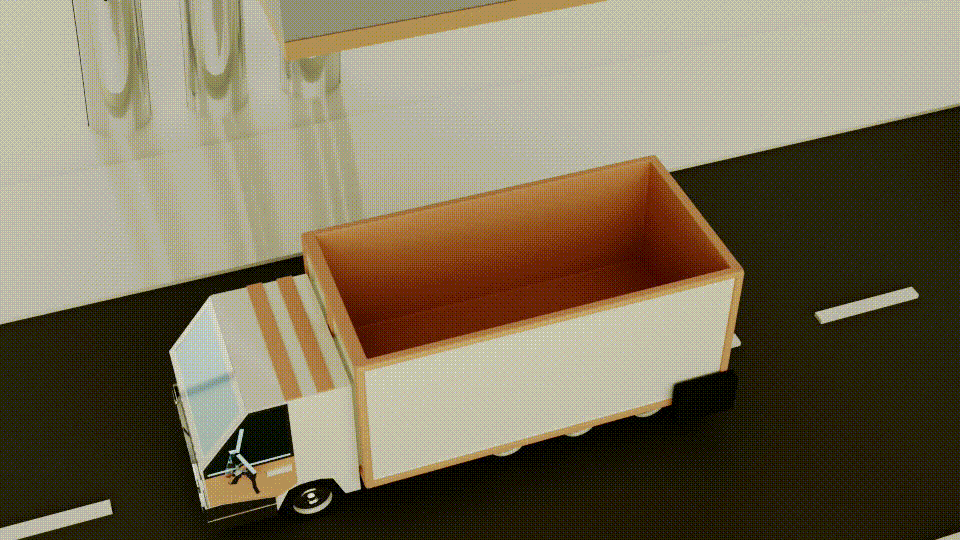
SHIPMENTS MANAGEMENT
Which company has never had a shipment problem? The more complex the shipping lane is, the more problems can arise and result in dramatic situations with a snowball effect. ML can bring an interesting insight in this processes, identifying key features combining multiple actors. This allows for durable optimization of the lane by the elimination of useless steps or even by combining shipments together. Finally, the most usable insight is to predict the starting date of the shipment based on the different actors, so that it may safely arrive in due time.
CUSTOMER RELATIONS
Last but not least, injecting ML in Customer Relationship Management allows to reduce the number of employees assigned to reporting the problems encountered at the end of the Supply Chain. Companies loss in terms of returned goods are tremendous and most of this information should be processed directly to influence the previous steps. Having Chatbots and automatic inspections at this last step can be of critical importance to help the ML Decision Making in the previous stages. Working towards greater client satisfaction in this way allows to directly correlate their problems to the practical aspect of the Supply Chain with the help of data from the communications between these algorithms.
All these facets of the Supply Chain Management and the way Machine Learning can improve their efficiency already give an overlook of what can be done, but having these communicate together can result in a perfectly automated manager. It can then correlate factors from the very beginning to the end of the lane, define which are the problems to solve, with an easy solution to implement and an analysis of the different SC components, allowing to discard the impractical actors or processes.