Data Governance
Our client, the Newton* company must keep more than 300’000 clients engaged throughout the country. The company sends, monthly, multiple commercial communications, as well as a magazine, whose elaboration and distribution is costly. The client database is the result of the fusion of different legacy databases, and thus the product of many compromises, which has created many duplicates.
After the use of professional duplicate finder software, which works using field matching, obvious duplicates have been merged. However, there remains more than 60’000 duplicates, whose fields cannot provide enough information to ensure the pairs found aren’t clients with the same name. These remaining duplicates cost more than 200’000CHF per year in superfluous mailings.
For a human being, identifying likely homonyms is a common ability. However, business as usual at the company translates into a regular increase in duplicates. Thus, the Newton company has to hire a worker whose sole activity is to find and remove duplicates. Such an endeavor is time-consuming: only 400 duplicates are processed each day, which comes down to a cost of almost 1CHF for each duplicate removed.
PEACOCK implemented a solution that combines the human ability to easily recognize probable homonyms with the speed of a computer. The solution, on the night of its deployment, immediately processed the entire database and identified 52’000 duplicates, reducing the number of uncertain homonyms to 8’000.
To achieve this feat, PEACOCK used neural network technology, deconstructing the database fields so that they could be fully exploited by the algorithm, so that, in turn, it could imitate the recurring patterns that give humans their ability to identify duplicates.
This problem, present in most databases, is a hindrance for companies wishing to take full advantage of new technologies, as well as a source of unnecessary expenses.
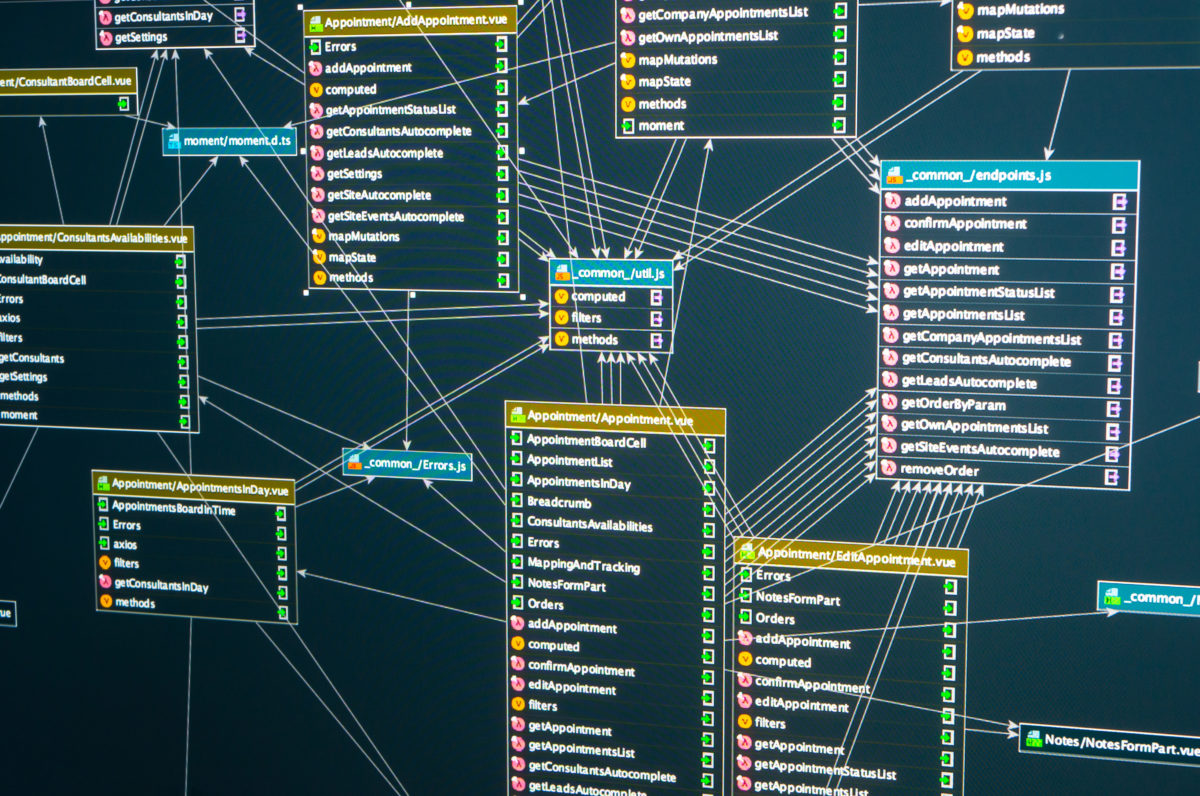
Interested in upgrading your database system?
Contact us:
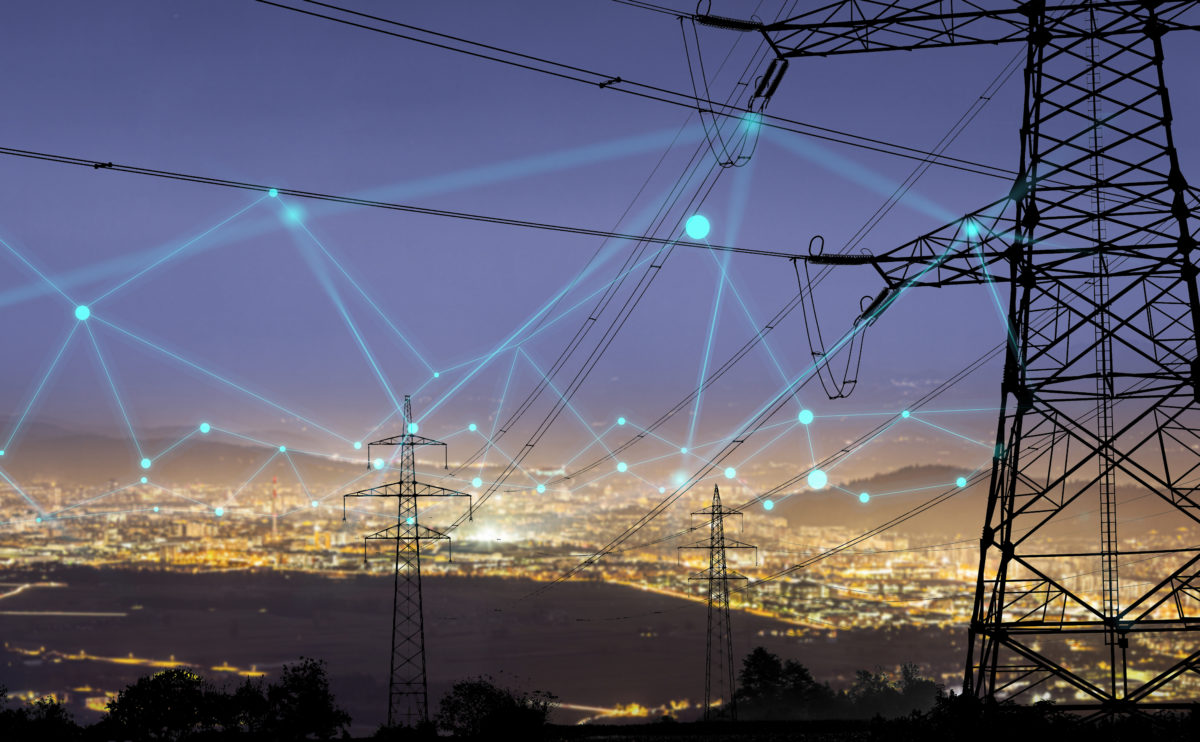
Ready to reduce your energy costs?
Contact us:
Energy Management
The Faraday* company operates in a location that combines a large surface area with a large volume. In addition, numerous energy installations are scattered on the site. This complex network suffers from a lack of efficiency, due in part to the opaque nature of the installation, as well as its gradual conception. Network loss points are difficult to identify and consumption estimates are therefore imprecise, despite good planning for future events, resulting in energy costs that are difficult to budget.
PEACOCK was consulted to model and then optimize this consumption. One of our teams of physicists worked, after inspection of the premises, to identify the points of consumption and analyse the partial energy readings held by the Faraday company.
The preliminary analysis revealed that it was difficult to approach the problem in any other way than statistically: the implementation of a system for measuring consumption by post is inefficient and costly. Moreover, all optimizations could only be due to a better allocation of resources. Faraday already optimised these allocations to the best of their ability, but the complexity of the network makes it difficult to assess the gains. If known, these gains could be used as a strong communication point for their policy of achieving a neutral energy balance.
Following the study, PEACOCK proposed a solution based on a physical design of the unique attributes of this network, using neural networks for the optimization of the specific applications of the model. The network trained on the consumption patterns of the previous decade, with the aim of making the model applicable over the longest possible duration.
This model suggests gains up to 30% of current consumption, decreasing related costs by the same amount.
Supply Chain
The Galileo* Company specializes in coffee imports and wishes to automatize its supply chain, and at the same time, reduce related costs. Its supply chain spans the whole globe: from South America to Italy, with a detour through Singapour. Due to the reach of the company’s activities, mishaps often happen. The Galileo company would like to be able to take preemptive steps to avoid them.
PEACOCK was mandated to evaluate the situation of the supply chain, and put forth a solution reducing delays caused by local setbacks. Indeed, due to a major occurrence affecting one of its supplier, the Galileo company has seen a large amount of its merchandise stuck in transit, which, in turn, has stopped its entire production chain. The company has thus made the choice to diversify its supplier, and entrusted PEACOCK with the responsibility to find prouction lines which minimize stoppage probability, through automated allocation of supply calls and product processing.
The solution evaluates the fiability of each actor and optimizes supply calls, taking into account all other parties, adapting in realtime to perturbative events in the supply ecosystem. The algorithm manages environmental, political and economical factors, in order to draw up a production plan which is optimized in regard to the current supply environment, optimized for path length and costs.
The algorithm is built to learn without any other supervision, in order to remain relevant every production cycle. The dependence on a supply chain manager’s particular experience is minimized, allowing easier knowledge transfer.
Machine learning has allowed, in this situation, to build a solution taking into account a large amount of data, granting the ability of constant learning, linked to the possibility to indentify interruptions in a network which evolves in time and space.
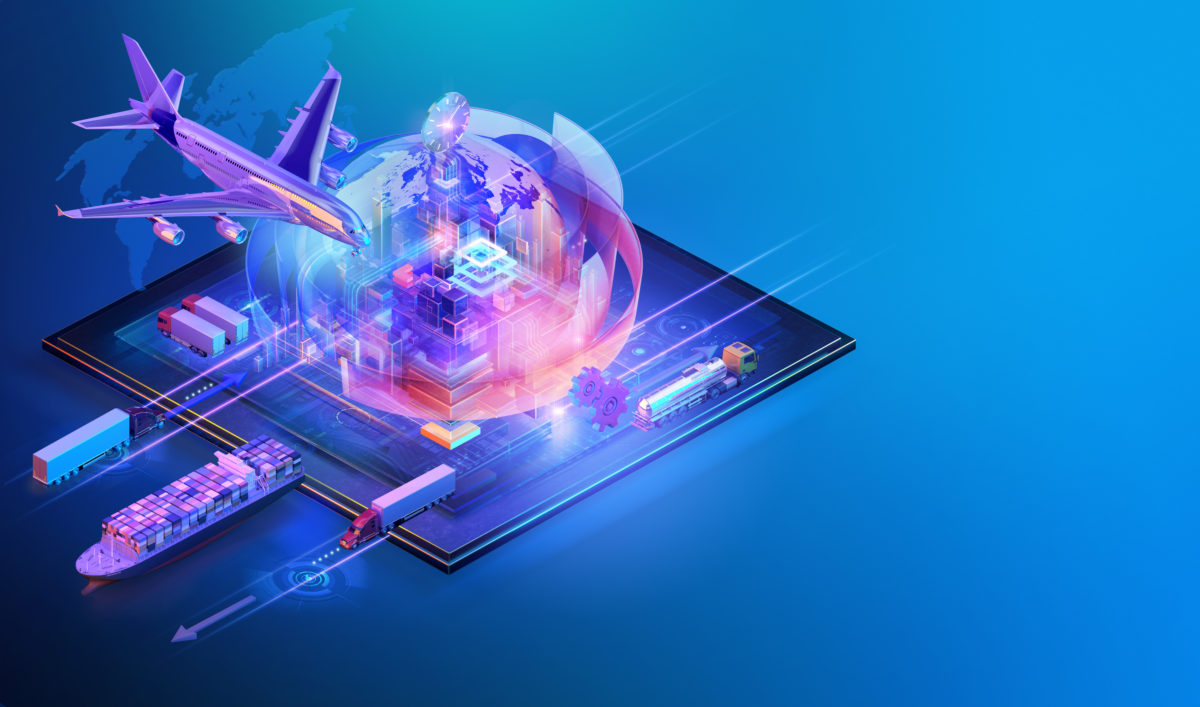
Curious about optimizing your Supply chain?
Contact us:
*Alias names